What is Synthetic Data?
Synthetic data is information artificially generated through computer simulations or AI algorithms, rather than being collected directly from the real world. It replicates real-world data conditions, making it suitable for training AI/ML models.
Unlike real-world data, which can be limited and difficult to obtain, synthetic data offers complete control over variations and scenarios that can be simulated, such as 3D images, realistic textures, or even the creation of specific situations that would be rare or expensive to capture in the physical world.
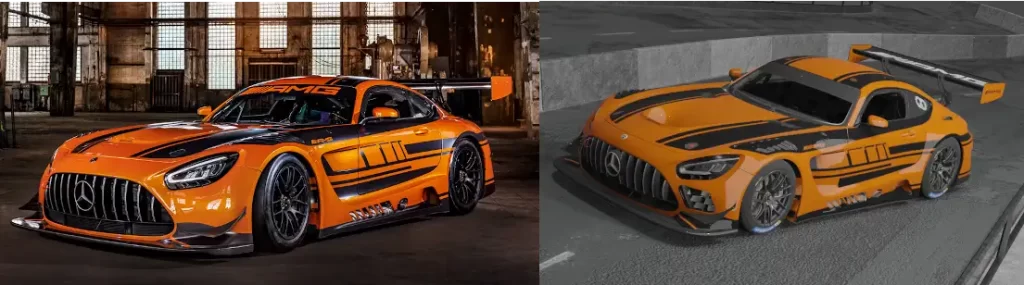
Why is Synthetic Data the Future of Computer Vision?
Synthetic data is changing the game in computer vision due to its numerous advantages. It is easier to generate at scale, can be adjusted to cover complex or rare scenarios, and avoids issues such as privacy concerns and bias found in real-world data. In industries like healthcare, manufacturing, and transportation, this flexibility is crucial.
In addition, synthetic data helps solve critical problems such as:
- A scarcity of real-world data for training complex models.
- High costs for capturing and annotating large volumes of data.
- Privacy concerns, especially in sensitive areas like healthcare.
Practical Use Cases for Synthetic Data
Synthetic data is being applied across various industries with promising results. Here are a few examples of how it is being used to solve real-world problems:
- Agriculture: Using synthetic data to count fruit on trees, detect diseases in seeds with hyperspectral imaging, and monitor soil anomalies.
- Healthcare: Analyzing medical images, such as tumor detection, where hyperspectral data helps identify patterns invisible to the naked eye.
- Transportation: Simulating traffic environments and creating annotated datasets to train autonomous vehicles.
Advantages of Synthetic Data Generation
By using synthetic data, companies can access benefits such as:
- Complete control over simulated scenarios.
- Cost savings compared to real-world data collection.
- Greater scalability for training models in complex and varied scenarios.
Moreover, the ability to generate accurate data on demand allows businesses to adjust their datasets as the model evolves, avoiding rework or dependence on external data sources.
At SynthVision, we collaborate with our clients to create synthetic datasets tailored to the needs of each project. We offer complete solutions, from data creation to the development of customized models that surpass the limitations of real-world data.
Your benefits when working with SynthVision include:
- Total flexibility in data generation.
- Development of complete AI/ML solutions.
- Specialized support in computer vision and synthetic data.
This was just a brief overview of how synthetic data generation is revolutionizing the training of computer vision models. Stay tuned for future posts with more real-world use cases and tips on how you can leverage this technology.
If you have any questions or suggestions for future posts, leave a comment!